written by: sorina andrei
graphics by: irene zhao
Reality is a subjective experience formed by our interpretations of past and present sensations. It is still a mystery, however, as to how we make sense of our reality. While each person may view the world differently, one theoretical model proposes a universal method that every human employs when making sense of things. How we understand and interact with reality depends on the intersection of math and cognition.
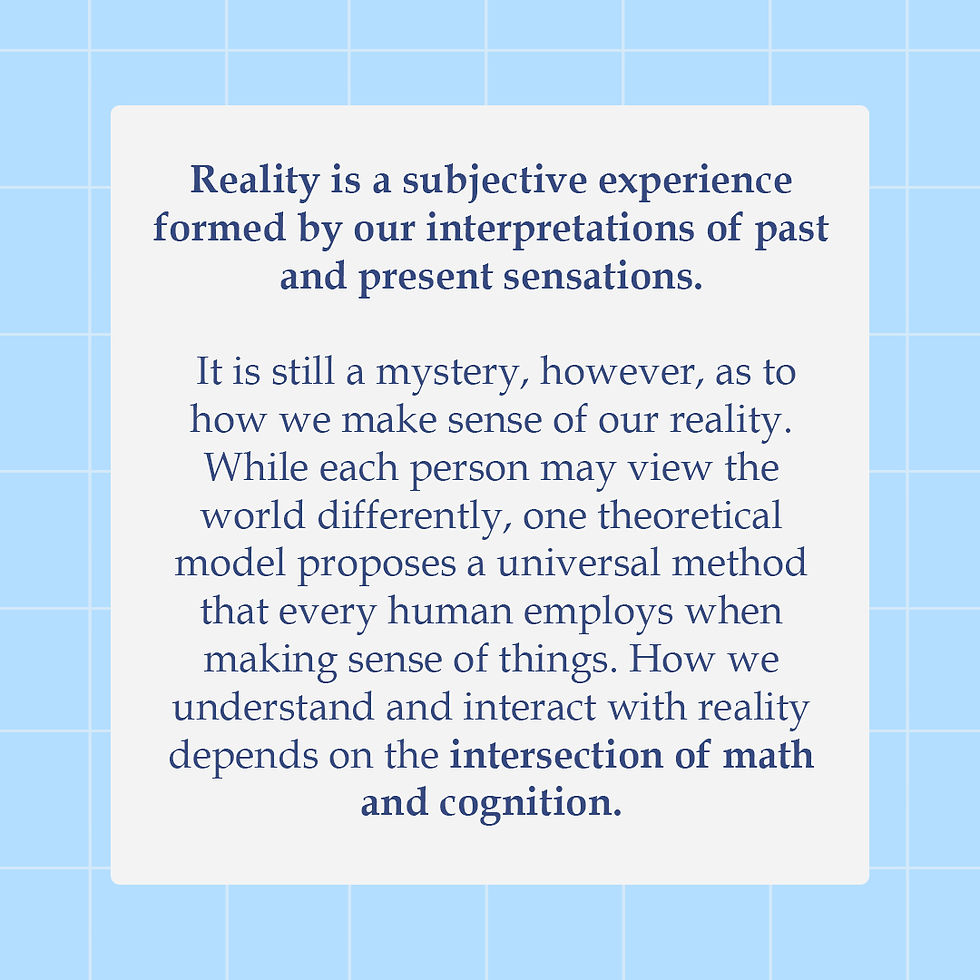
In the mid-1700s, Reverend Thomas Bayes developed a formula, known as Bayes’ theorem (1). Bayes’ theorem describes the probability of an event occurring based on prior knowledge of the conditions related to the event (1,2). As more information or evidence of the conditions become available, the theorem is updated to form a more precise probability of the hypothesis. This process of deduction is called Bayesian inference.
Bayesian inference computes the posterior probability of an event—the probability of an event after accounting for the relevant information of the conditions (1,2). Specifically, what is the probability of event A occurring given that event B took place?
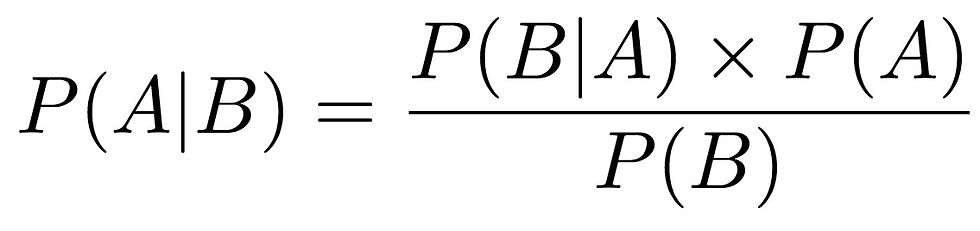
P(A|B) = the posterior probability (the probability of A given B, after B is observed)
P(A) = the prior probability (the probability of A occurring before considering the probability of B)
P(B|A) = the likelihood function (the probability of B given A)
P(B) = the marginal likelihood (the probability of B without considering A)
Mathematically, the posterior probability is a consequence of the prior probability and the likelihood function (1,2). The greater the accuracy of a prior belief, the greater the posterior probability will be influenced by it. Ultimately, we make an inference according to the interplay between prior knowledge and new evidence.
We make use of the Bayesian model everyday to make deductions and decisions. Say that you ordered food from a new restaurant and later that day you get food poisoning. Given that the food was the only thing in your diet that changed recently, you deduce that the probability that your sickness is due to the food is higher than the probability of something else causing it. If you order food from that restaurant again and still get food poisoning right after, the probability that the food is behind your sickness is even greater because you have more evidence confirming this relationship.
Bayesian inference is how we learn from our experiences. An individual uses their prior knowledge to make connections between events, form conclusions, and plan for the future. Now you know to avoid that restaurant if you want to prevent getting food poisoning again.
How we perceive the world is also dependent on Bayesian inference. Perceptual images are the product of expectations of previous experiences and present sensations (3). To gain an accurate perception of reality, it’s essential for this process to function properly with the other mechanisms in the brain. Many perceptual problems often involve abnormalities in Bayesian inference strategies (4,5). Certain symptoms of schizophrenia, for example, can be explained through the lens of Bayesian inference (4–6).
Schizophrenia is a mental disorder that is characterized by abnormal interpretations of reality (4). Hallucinations are a symptom of the disorder in which individuals perceive things that aren’t actually there (like hearing voices). Many researchers hypothesize that Bayesian inference plays a role in the formation of these hallucinations. When small perceptual illusions or biases occur, the brain will try to make sense of what it is perceiving and update its system to integrate this new information. The update increases the prior probability of these illusions happening again in the future, resulting in stronger biases for such perceptions. As these abnormal percepts occur more frequently, the expectation of them occurring again increases as well. Eventually, this spiralling effect leads to the complex hallucinations typically associated with severe cases of schizophrenia (4).
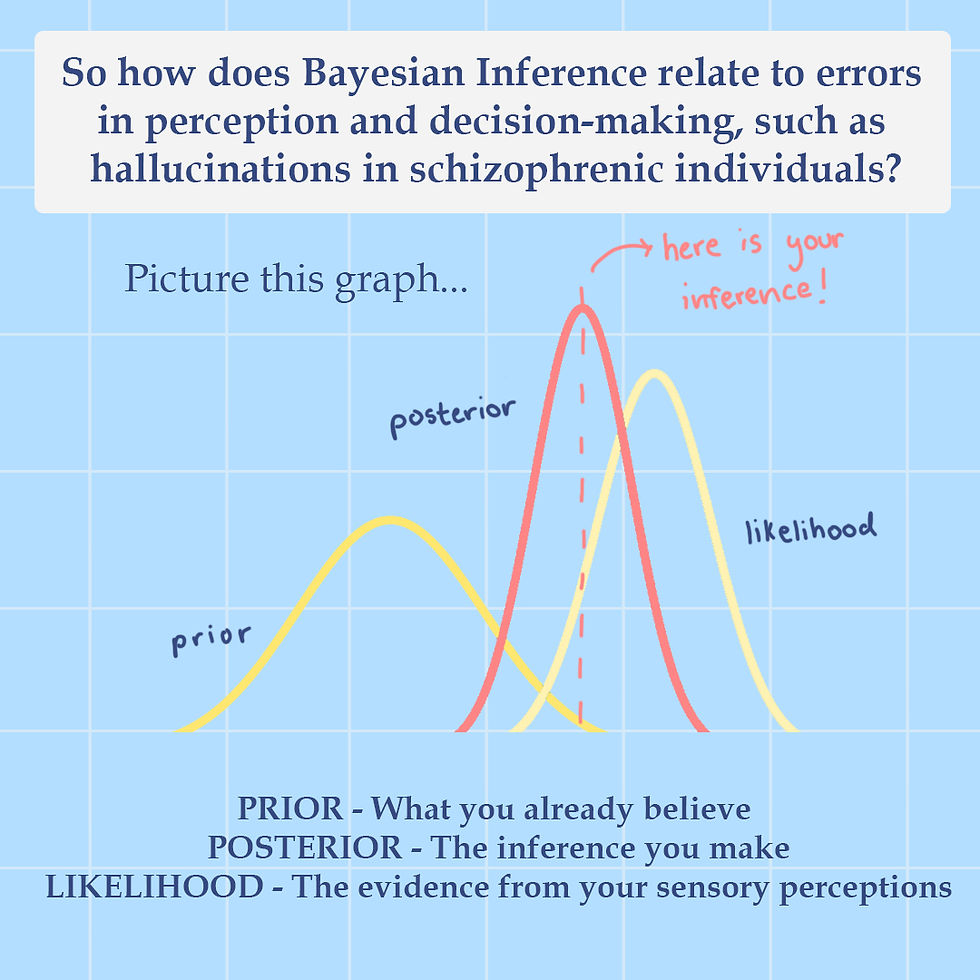
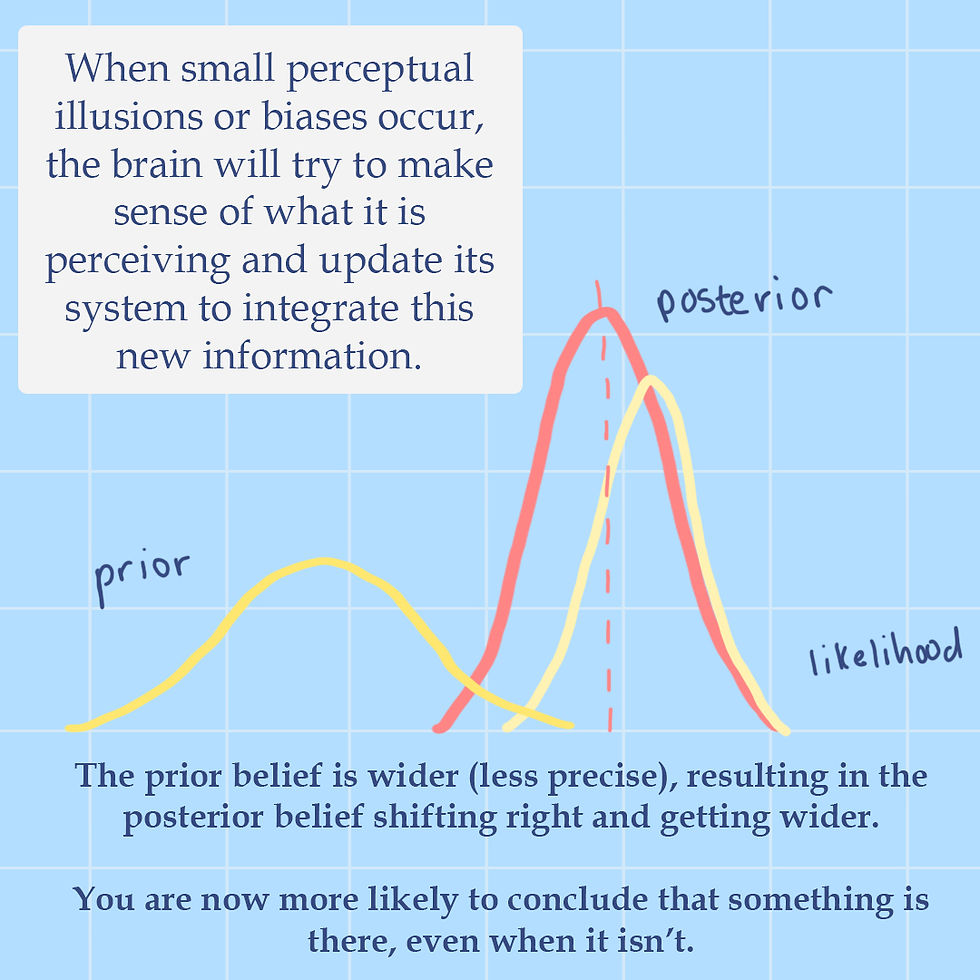

Delusions are another symptom of schizophrenia that have been studied using Bayesian inference (4–6). These rigid, false beliefs about reality result from incorrect inferences that deviate from the Bayesian model. Individuals with schizophrenia-related delusions tend to jump to conclusions, engaging in hasty decision-making more frequently in comparison to those without the disorder (6,7). In several experiments observing this behaviour, researchers found that patients with delusions require less evidence to make firm decisions and that they are often confident in their choices. This bias to jump to conclusions can result in delusions, as the Bayesian model to form accurate perceptions is not followed. Despite having insufficient prior knowledge about an idea or concept, individuals with delusions strongly hold onto their false beliefs about reality (6,7).
Not all cognitive and behavioural patterns follow the Bayesian model. Even without the presence of mental disorders, humans are unpredictable and spontaneous creatures that aren’t bound by the limits of a mathematical theorem. While Bayesian inference cannot account for every choice or perception we make, it allows us to gain a better understanding of how we make sense of the world.
References
3. Goldreich D. Bayesian Brain. Lecture presented at; 2021; McMaster University.
Comments